Here's Why Our Gleaming Self-Driving Future Has Been Delayed Indefinitely
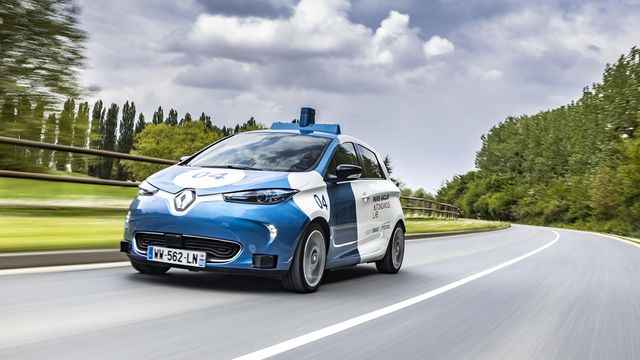
It wasn't long ago when we heard bold predictions of our robotaxi future, promised to arrive by 2020 or shortly thereafter. Now, a quick survey of autonomous tech developers that were aiming for Level 5 just a few years ago reveals slashed budgets, scaled-down goals and a much more skeptical tech landscape.
The previous decade started out carefully enough when it came to autonomous vehicle research, with a number of developers cautiously revealing prototypes of Level 2 and Level 3 semiautonomous vehicles that, depending on who you asked, were either decades or years away. Autoweek attended a great number of these demos in the early part of the decade, and watched as developers and CEOs hedged and carefully promised everything ranging from a revolution in driving in mere years to some form of narrow use of autonomous tech in the far future once the legislative framework emerged from the dark ages and allowed for some nationwide uniformity.
Those early years were largely marked by intense skepticism toward being permitted to do anything at all by various state governments, which jockeyed for position to craft either the most permissive or the most restrictive regulations pertaining to autonomous vehicles, egged on by Silicon Valley. Those early efforts were also marked by a very sober assessment of the chances of even Level 2 vehicles being allowed on the roads within the next two decades—"maybe decades, maybe never" was a common refrain we heard as suppliers and manufacturers gave us glimpses of mockup roads and towns on proving grounds and even out on the very real autobahn. A Level 2 future, where every car would have some sort of highway autopilot feature that allowed drivers to take their eyes off the road for a few minutes at a time, seemed within reach, as long as one or more jurisdictions permitted it.
That era, stretching from 2007 to roughly 2014, is what is now viewed as the early years of the development of autonomous driving tech, and it was followed by a much more exuberant but shorter period stretching from 2015 till 2018, when Level 5 autonomy seemed close enough on the horizon to be visible, like a mirage in the desert, but somehow still out of reach.
These heady years, now far in the rearview mirror, were characterized by an unusual level of enthusiasm as pallets of cash were fed into autonomous startups by Silicon Valley and automakers, in a version of the 1960s space race. The mad rush was seen through a winner-take-all lens, in which a single winning developer would reap all the monetary benefits of its Level 5 technology by patenting it and by dominating the market in a near-monopolistic fashion. This approach was spawned by similar winner-take-all efforts to disrupt some industry, notably the taxi industry, which in similar fashion was rebranded as the sphere of "personal mobility solutions." As soon as some developer perfected the software and hardware well enough, talking heads on YouTube and TV programs told us, a driver-free utopia would spontaneously arrive where we would simply nap on the way to work as our cars did the driving.
That era of Level 5 optimism , which largely ended two years ago, was fueled by a belief that getting from Level 3 to Level 5 would take just as many years as it took to get from Level 1 to Level 3—a fallacy called out at the time by relatively few skeptics—and was to a great extent boosted by prototypes that seemed to be able to drive in complex traffic without disengaging or crashing into anything, "proven" by YouTube videos posted by their developers. Indeed, Uber, among a number of leading developers, had racked up many autonomous miles with its fleet of modified Volvo test vehicles in several cities, before the unfortunate death of Elaine Herzberg in the spring of 2018. Herzberg became the first pedestrian to be killed by an autonomous test vehicle. Uber suspended its testing program in response, even as Tesla Autopilot accidents continued to stack up, in turn sending a signal to the industry that Levels 4 and 5 may take a much longer time to sort out, and that self-driving cars may not be just around the corner. Early 2018 also marked a point in time when autonomous developers appeared to acknowledge that completely driverless vehicle operation with humans on board could indeed be decades instead of years away, while pressing on with lower levels of autonomy.
But reliable semiautonomous capabilities above Level 3 that could be safely introduced to cars that people could actually buy, irrespective of the regulatory landscape, seemed to hit a wall. Within the last two years a number of developers (but not all) have scaled back: Magna and Lyft have parted ways on autonomous development, while Audi has given up on plans to offer even Level 3 autonomy in the current A8 sedan. Various other developers, with the major exception of Mobileye, have adopted an agnostic stance on just when something resembling Level 4 could be rolled out, even as trials of the technology continue.
What brought about the current era of Level 5 skepticism and a reconsideration of what's possible?
A combination of software and sensor hardware limitations, as well as very real problems with our 20th century infrastructure that now seem intractable, even in the most ideal of conditions.
Starting first with sensors, autonomous developers have recognized that even an unlimited number of cameras, radar and lidar sensors—as many as can fit on the roof and bodywork—still paint a more limited picture of a car's environment than what humans can perceive visually, despite producing far more visual data for software to interpret, something that had earlier guided autonomous vehicle development. If what the human eye sees could be correctly interpreted by software and fed into a computer, some early developers suggested, then a car could safely navigate an environment. This view appeared to be echoed by Tesla, which used an array of cameras and radar but shunned lidar, positing that it already had all the hardware needed to observe the environment and that the needed software could be developed and uploaded later via over-the-air updates.
But a number of other developers seemed to recognize that human drivers, in reality, identify and respond to objects much farther ahead than permitted even by the current radar, cameras and lidar, effectively making plans in response to things happening half a mile ahead or even farther. For example, a lidar sensor can certainly paint an accurate-enough picture of the vehicles and buildings a few dozen yards away from the sensor, but correctly sensing and classifying objects several hundred yards away—such as a parked fire truck, to use a common Tesla foe—is effectively out of range, at best. In what scenario might you need to see a fire truck doing something half a mile down the road? Usually when it's parked in the road and is assisting a disabled vehicle. Human drivers can spot such hazards from remarkable distances, at times, and can take action simply by moving over a couple lanes and slowing down, as other vehicles do the same. However, the software to recognize and correctly identify such items from a distance is not quite there yet, and neither is the processing power to observe and track all objects over a tremendous distance.
Staying with the same scenario, one could say, "Well, all the hardware requires is a camera that can identify solid objects, the road surface and markings half a mile down the road." This is still negated by complex movement in something as minor but commonplace as an accident zone on the freeway: The fire truck may be stationary or moving slowly, but it could be signaling that it's about to move a lane or two over to the right, which could necessitate a lane change by the autonomous car while still several hundred yards away. Can the software, which is now tasked with interpreting images from a forward-facing camera, see the fire truck's directional blinker and respond to its sudden desire to move over in time? A human driver might, out of an abundance of caution, but can current software make decisions about a fire truck's directional blinkers from a quarter of a mile away?
A similar scenario involves approaching emergency vehicles that can be heard but not seen. Human drivers might slow down when hearing a siren but without seeing any emergency vehicles, but autonomous vehicles that can identify siren sounds and take evasive action will still need to be able to distinguish between vehicles approaching in oncoming traffic on a divided highway, in which case no action needs to be taken, and an unseen emergency vehicle approaching from behind. Likewise, certain vehicles like school buses require a degree of visual nuance from autonomous vehicles. Is the school bus merely parked on the side of the road, or is it stopped and has deployed its pivoting stop sign while unloading students? These are questions that Level 5 autonomous vehicles will need to be able to get right a pretty high percentage of the time.
If these scenarios seem purely hypothetical or a high bar at the moment for the current sensor suites and hardware, recall the fact that highway scenarios have been viewed as a much more solvable landscape for vehicles with advanced levels of autonomy: All cars are moving in the same direction, all the lanes are (mostly) clearly marked, and there is usually enough time to react to surprises.
Urban environments pose a much greater challenge to sensors and software alike, and they are ultimately the environment where their limitations can be readily observed. In an urban landscape a human driver can see and respond to a much greater number of potential hazards—including poor signage, complex intersections, unpredictable cyclists and pedestrians, and traffic lights—than a camera or lidar can. For example, a pedestrian can be obscured from a camera or lidar by a minivan parked to the right of your lane of travel—you can see that pedestrian, who wants to dart into traffic, through the rear and front windows of the minivan, but none of the sensors can because he or she is too hidden. Or if the parked vehicle is completely opaque to the driver, like a brown UPS van, you can still see the pedestrian's head peering out from behind the parked van, and you can make the decision to slow down, move over a lane or flash your lights to let them pass. Can current systems in testing detect such hazards and make appropriate decisions regarding the intentions of pedestrians who may or may not be completely visible a high enough percentage of the time?
These are the aspirational qualities of the current sensor hardware, as well as software, ones that make the simulated urban environments used in proving grounds by automakers necessary. And they also touch upon the second major issue faced by autonomous developers: the road environment itself.
Very early on, highways were seen as the ideal environment for Level 2 and 3 semiautonomous vehicles due to their lack of stop lights and (for the most part) clear lane markings. Signage and the use of turn signals by other vehicles, ones close enough to the semiautonomous vehicle in question, were seen as a secondary priority: The semiautonomous vehicle would know where it is thanks to GPS, and the movements of other vehicles could be sensed by cameras, radar and lidar. This is ultimately the environment for which Tesla designed its semiautonomous Autopilot driver assist system, relying upon cameras and radar (but not lidar) to monitor the car's position using lane markings and GPS.
The limitations of this approach became painfully obvious even before the first accidents that may have been caused by incorrect lane marking interpretation materialized. The first driver death linked to limitations of Autopilot occurred in May 2016 when a Model S driven by Joshua Brown collided with a semitruck making a legal turn on a highway in front of the Model S. The Tesla effectively drove under the truck's trailer, which ripped off its roof, and continued to travel for several hundred feet. The NTSB report of the crash made the following finding, among others:
"The Tesla’s automated vehicle control system was not designed to, and could not, identify the truck crossing the Tesla’s path or recognize the impending crash," the NTSB said in its report. "Therefore, the system did not slow the car, the forward collision warning system did not provide an alert and the automatic emergency braking did not activate."
While the circumstances of the crash and the tech involved were a cautionary tale of overreliance on technology—something the NTSB noted, as well—they were also a reflection of the insufficient hardware and software on board. This particular case, in addition to several subsequent accidents, highlighted the limitations of hardware and software that itself is now a bit dated, in a road environment that was close to ideal. Perhaps it's correct to treat Tesla's Autopilot as an isolated case—the system was not meant to offer anything above Level 2 and requires constant driver attention—were it not for the company's insistence that Full Self-Driving, or FSD, would rely on the same suite of sensors, which are already being fitted to production cars.
Ultimately, Tesla's claim of being able to deliver Full Self-Driving with the current suite of sensors reveals something else about sensor hardware: Even the most perceptive sensor suite in a hypothetical Level 5 vehicle would need to be able to handle a much more complex urban environment, and to respond not only to pedestrians, cyclists, motorcyclists and other vehicles, but also complex traffic lights and lane markings in various light conditions and with various complicating factors, such as being forced to drive behind a bus. Level 2 prototypes have certainly shown the ability to follow other vehicles, but their ability to navigate from behind something and respond to objects not within their line of sight is an ability that's only now being developed.
This addresses another gap in the current road environment: lack of uniformity in signage, lights and traffic patterns. If a Level 5 vehicle would have any hope in an urban environment, the on-board sensors would need to be able to paint a very detailed picture of the road space, prior to taking into account the complex movements of all the other road users. Putting road quality aside for a moment, the mere issue of road design has been causing endless headaches for testers of autonomous vehicles in real street environments. Autonomous vehicles have to contend not only with erratically painted lines, but the presence of curbs on medians, which could be struck by a vehicle even following the painted road markings.
Lately, autonomous developers have turned to computer environments for testing, allowing software to cover millions of miles in a much shorter span of time and without risk to road users. But even this approach, while being useful to train the software on something resembling real roads, does not quite solve the problem.
The technical limitations and road scenarios we've listed above paint Level 5 as being vaguely possible on a technical level decades down the road, once more advanced sensors are developed. But in the meantime, this hasn't stopped automakers from aiming for Level 4 autonomy in a geofenced area, set apart from Level 5 by certain other conditions. The companies currently aiming for Level 4 imagine either certain robotaxi applications or use in cargo trucks—there are plenty of developers currently aiming for these two supposed cash cows—even though concerns about costs and real-world application remain.
Are we in for a very long era of Level 3 and 4 autonomy in narrow applications and environments?
This appears to be the case for now, until sensor technology overcomes a few barriers while also becoming cheaper to produce, and until software can become "safe enough," which is what the bar has been lowered to. Meanwhile, other concerns remain with the business side of higher levels of autonomy. Considering robotaxis first, it has been clear for a long time that the current and apparently still unprofitable ride-sharing apps would like to get rid of human drivers in their taxis altogether. But despite pouring billions into autonomous development to get rid of the cost of paying a human they haven't quite figured out who will bear the cost of the autonomous components, due to a still-high price of the sensor tech, and assuming the software itself was up to the task.
Likewise, cargo carriers have also been enamored with the idea of not paying drivers, but until completely driverless trucks become a reality—itself perhaps a mirage on the horizon, decades away—they still have to pay drivers to get the trucks on a highway where some kind of Level 3 or 4 system can be engaged to presumably make the driving part easier.
On a consumer level, meanwhile, Tesla has proven that some buyers will spring for a Level 2 system that requires constant driver attention—itself a mirage in a way—lest the system misread some lane markings and send the car into a concrete barrier in a tiny percentage of cases. Effectively, this has proven that drivers are willing to roll the dice on a system that's good enough, while accepting some risk of a catastrophic failure all for the convenience of not having to be alert at all times while driving—and are willing to pay for it. Whether that's an encouraging factor for autonomous tech developers and those seeking to profit from it remains to be seen.
출처 : https://www.autoweek.com/news/technology/a32782600/why-level-5-autonomous-driving-has-not-happened/